ADVANCED HBA1C PREDICTION MODEL FOR PROACTIVE DIABETES MANAGEMENT
LICENSING & PARTNERING OPPURTUNITIES
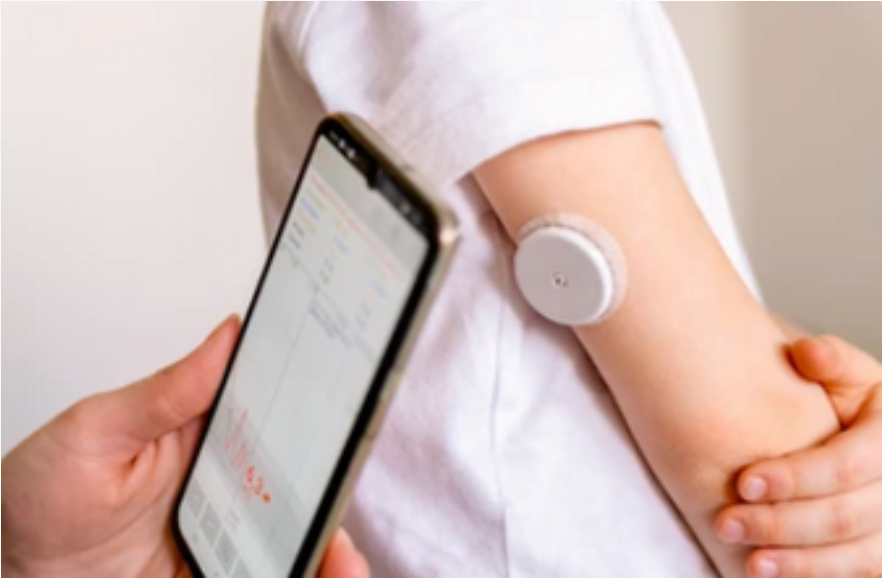
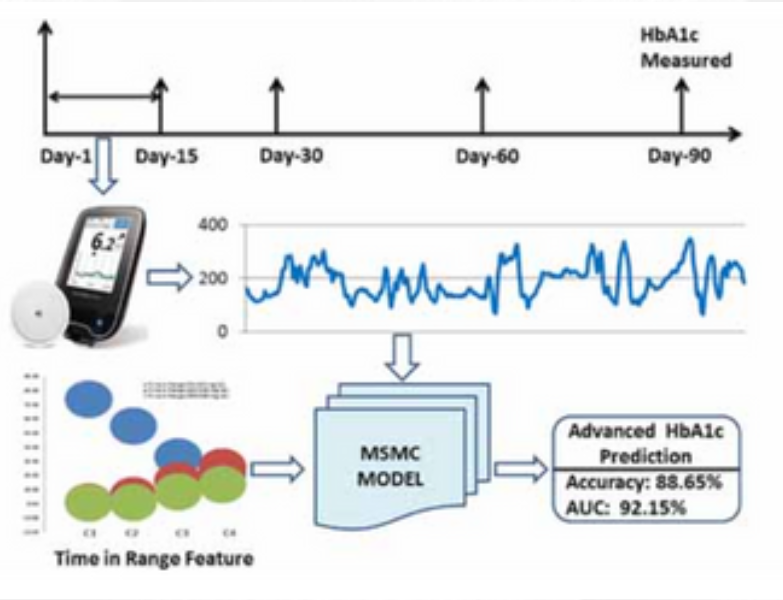
This invention introduces an advanced HbA1c Prediction Model designed to revolutionize diabetes management. Developed by a team of distinguished professionals, this innovative model leverages time series CGM sensor data, convolutional neural network (CNN), and few-shot learning (FSL) techniques for accurate and proactive HbA1c level prediction. The model demonstrates an unprecedented accuracy of 92.30%, marking a significant milestone in the realm of diabetes care.
The Problem
The glycated hemoglobin (HbA1c) serves as a critical biomarker for diabetes management. While regular monitoring is essential, the absence of an advanced prediction model has hindered proactive interventions. Elevated HbA1c levels pose risks of diabetes-related complications, emphasizing the need for early predictions to facilitate timely adjustments in treatment plans and lifestyle. Traditional approaches lack the accuracy demanded for such predictions, necessitating a novel solution.
The Solution
The Advanced HbA1c Prediction Model pioneers the conversion of time series CGM sensor data into binary and histogram images. These images undergo feature extraction through a convolutional neural network (CNN) employing few-shot learning (FSL) techniques. The normalized FSL-distance (FSLD) metric is introduced for precise image differentiation based on HbA1c levels. The final prediction is accomplished using a k-nearest neighbor (KNN) model with majority voting, achieving an unprecedented accuracy of 92.30%
APPLICATIONS
- Proactive Diabetes Management: Enable early interventions and adjustments in treatment plans based on advanced HbA1c predictions.
- Reduced Risk of Complications: Lower HbA1c levels contribute to a decreased risk of diabetes-related complications, emphasizing the importance of accurate predictions.
- Patient and Physician Empowerment: Equip patients and physicians with the tools for informed decision-making and personalized diabetes management.
- Unprecedented Accuracy: Achieve a groundbreaking accuracy of 92.30% in predicting HbA1c levels, surpassing existing models in the field.
PATENT STATUS
US & Qatar Patent Applications have been filed for this invention
COMMERCIALIZATION OPPORTUNITY
Sidra Medicine & HBKU are offering this technology for licensing or industrial collaboration on tech maturation. For more information, please contact: TechTransfer@sidra.org